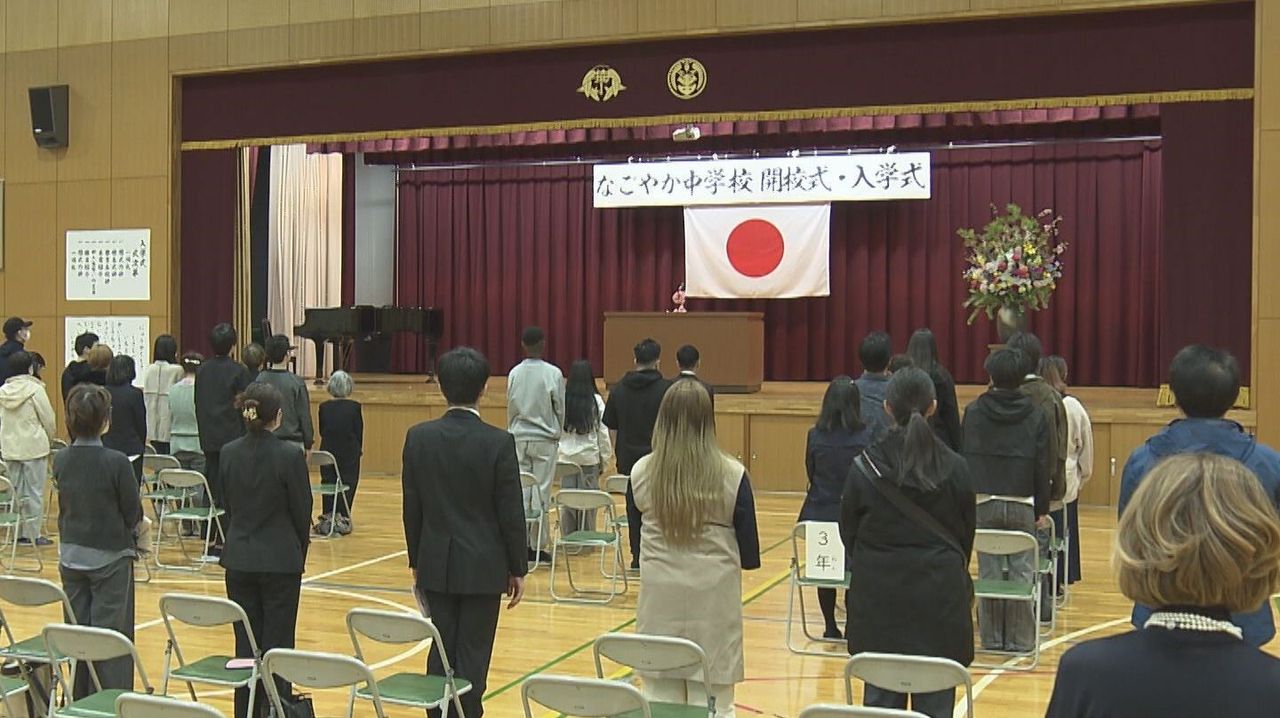
88歳の新入生も 名古屋に夜間中学
Source link
Views: 0
昨年10月からGitHub Copilotの使用状況を取得できるAPIが提供されました。
組織の管理者権限を持つアカウントのアクセストークンじゃないと取得はできないようになっています。
APIからは以下のデータが取得することができます。
・過去28日間のデータ
・アクティブユーザーとエンゲージメントの高いユーザーの数
・GitHub Copilotのコード提案数、採用数、採用行数
・Copilot Chatの使用回数
・上記内容のIDE別、プログラミング言語別の内訳
GitHub Copilotが一般リリースされてから全社的に導入を推進しています。
しかしアンケートやヒアリングといった方法でしか導入効果と活用率が見えていませんでした。
APIを使って正確な情報まで取得できるので、Microsoft Fabric(データ分析基盤環境)を使って、社内の利用状況を可視化してみます。
自社の環境にFabricのリソースを作っておく必要があります。
※Microsoft Fabricは一番低いプランでも月5万円ほどかかります。
今回のデータ分析環境を作成します。
「新しいワークスペース」を選択
ワークスペース名を入力して「適用」を選択
データを保存するストレージを作成します。
左上の「新しい項目」から「レイクハウス」を選択
名前を入力して「作成」を選択
PythonでMetrics APIを叩いて情報を取得します。
左上の「新しい項目」から「ノートブック」を選択
先ほど作成したLakehouseを紐づけます。
「Add data items」から「既存のデータソース」を選択
Lakehouseを選択して「接続」を選択
access token
にはGitHub.comの設定画面からトークンを作成したものを、org
には自分が所属している組織の名前を入れてください。
import requests
headers = {
"Accept": "application/vnd.github+json",
"Authorization": "Bearer " ,
"X-GitHub-Api-Version": "2022-11-28",
}
response = requests.get("https://api.github.com/orgs//copilot/metrics" , headers=headers)
response_json = response.json()
情報が取得できました。
ちなみに普通にリクエストを飛ばすと28日分取得するので、日付を指定したい場合は以下のようにします。
例)前日の1日分だけ取得する場合
import requests
import datetime
from dateutil.relativedelta import relativedelta
today = datetime.date.today()
yesterday = today + relativedelta(days=-1)
headers = {
"Accept": "application/vnd.github+json",
"Authorization": "Bearer access token>,
"X-GitHub-Api-Version": "2022-11-28",
}
params = {
"since": yesterday
}
response = requests.get("https://api.github.com/orgs//copilot/metrics" , headers=headers, params=params)
response_json = response.json()
ここでAPIから取得できる情報の詳細を解説
日別に配列で情報を取得できます。
[
{
"date": "2024-06-24", # 日付
"total_active_users": 0, # アクティブユーザー数
"total_engaged_users": 0, # 利用ユーザー数
"copilot_ide_code_completions": { # IDE内でのコード提案に関する¥情報
"total_engaged_users": 0, # 利用ユーザー数
"languages": [ # 言語別に配列形式で格納
{
"name": "python", # 言語名
"total_engaged_users": 0 # 利用ユーザー数
}
],
"editors": [ # IDE別に配列型式で格納
{
"name": "vscode", # IDE名
"total_engaged_users": 0, # 利用ユーザー数
"models": [ # モデル別に配列型式で格納
{
"name": "default", # モデル名(defaultは通常のオリジナルモデル)
"is_custom_model": false, # カスタムモデルか
"custom_model_training_date": null, # いつカスタムさせたか
"total_engaged_users": 0, # 利用ユーザー数
"languages": [ # モデル内で言語別に配列型式で格納
{
"name": "python", # 言語名
"total_engaged_users": 0, # 利用ユーザー数
"total_code_suggestions": 0, # その言語で提案した回数
"total_code_acceptances": 0, # その言語で提案したコードが採用された回数
"total_code_lines_suggested": 0, # その言語で提案したコードの行数
"total_code_lines_accepted": 0 # その言語で提案したコードが採用された行数
},
]
}
]
},
]
},
"copilot_ide_chat": { # IDE内でCopilot Chatの情報
"total_engaged_users": 0, # 利用ユーザー数
"editors": [ # IDE別に配列型式で格納
{
"name": "vscode", # IDE名
"total_engaged_users": 0, # そのIDEで利用したユーザー数
"models": [ # モデル別に配列型式で格納
{
"name": "default", # モデル名
"is_custom_model": false, # カスタムモデルか
"custom_model_training_date": null, # いつカスタムしたか
"total_engaged_users": 0, # 利用ユーザー数
"total_chats": 0, # チャット回数
"total_chat_insertion_events": 0, # 提案したコードが挿入された回数
"total_chat_copy_events": 0 # 提案したコードがコピーされた回数
},
]
}
]
},
"copilot_dotcom_chat": { # GitHub.com内でのCopilot Chatの情報
"total_engaged_users": 0, # 利用ユーザー数
"models": [ # モデル別に配列型式で格納
{
"name": "default", # モデル名
"is_custom_model": false, # カスタムモデルか
"custom_model_training_date": null, # いつカスタムしたか
"total_engaged_users": 0, # 利用ユーザー数
"total_chats": 0 # チャット回数
}
]
},
"copilot_dotcom_pull_requests": { # GitHub.com内でCopilotのPull Request機能に関する情報
"total_engaged_users": 0, # 利用ユーザー数
"repositories": [ # リポジトリ別に配列型式で格納
{
"name": "demo/repo1", # リポジトリ名
"total_engaged_users": 8,
"models": [
{
"name": "default", # モデル名
"is_custom_model": false, # カスタムモデルか
"custom_model_training_date": null, # いつカスタムしたか
"total_pr_summaries_created": 0, # 要約機能を何回使用したか
"total_engaged_users": 0 # 利用ユーザー数
}
]
},
]
}
}
]
テーブルは以下の11個に分けて、リレーションシップで相互関係を結ぶようにします。
ですので、テーブルに入れるための11個の配列を用意。
copilot_global_data =[]
copilot_ide_completions = []
copilot_ide_completions_languages = []
copilot_ide_completions_editors = []
copilot_ide_completions_editor_models = []
copilot_ide_completions_editor_model_languages = []
copilot_ide_chats = []
copilot_ide_chat_editors = []
copilot_ide_chat_editor_models = []
copilot_dotcom_chats = []
copilot_dotcom_chat_models = []
copilot_dotcom_pull_requests = []
copilot_doctcom_pr_repositories = []
APIから取得したレスポンスを上記11個の配列に追加していきます。
またリレーションを結ぶので、各配列ごとにuuidで一意のキーと親テーブルに値する配列のキーを持たせます。
import uuid
for entry in response_json:
global_id = uuid.uuid4()
copilot_global_data.append({
"global_id": global_id,
"date": entry.get("date"),
"total_active_users": entry.get("total_active_users"),
"total_engaged_users": entry.get("total_engaged_users")
})
completions = entry.get("copilot_ide_code_completions", {})
copilot_ide_completion_id = uuid.uuid4()
copilot_ide_completions.append({
"copilot_ide_completion_id":copilot_ide_completion_id,
"global_id": global_id,
"total_engaged_users": completions.get("total_engaged_users")
})
for lang in completions.get("languages", []):
copilot_ide_completions_language_id = uuid.uuid4()
copilot_ide_completions_languages.append({
"copilot_ide_completions_language_id": copilot_ide_completions_language_id,
"copilot_ide_completion_id":copilot_ide_completion_id,
"language": lang.get("name"),
"total_engaged_users": lang.get("total_engaged_users")
})
for editor in completions.get("editors", []):
copilot_ide_completions_editor_id = uuid.uuid4()
copilot_ide_completions_editors.append({
"copilot_ide_completions_editor_id": copilot_ide_completions_editor_id,
"copilot_ide_completion_id":copilot_ide_completion_id,
"editor": editor.get("name"),
"total_engaged_users": editor.get("total_engaged_users")
})
for model in editor.get("models", []):
copilot_ide_completions_editor_model_id = uuid.uuid4()
copilot_ide_completions_editor_models.append({
"copilot_ide_completions_editor_model_id": copilot_ide_completions_editor_model_id,
"copilot_ide_completions_editor_id":copilot_ide_completions_editor_id,
"model": model.get("name"),
"is_custom_model": model.get("is_custom_model"),
"custom_model_training_date": model.get("custom_model_training_date"),
"total_engaged_users": model.get("total_engaged_users")
})
for model_lang in model.get("languages", []):
copilot_ide_completions_editor_model_language_id = uuid.uuid4()
copilot_ide_completions_editor_model_languages.append({
"copilot_ide_completions_editor_model_language_id": copilot_ide_completions_editor_model_language_id,
"copilot_ide_completions_editor_model_id":copilot_ide_completions_editor_model_id,
"language": model_lang.get("name"),
"total_engaged_users": model_lang.get("total_engaged_users"),
"total_code_suggestions": model_lang.get("total_code_suggestions"),
"total_code_acceptances": model_lang.get("total_code_acceptances"),
"total_code_lines_suggested": model_lang.get("total_code_lines_suggested"),
"total_code_lines_accepted": model_lang.get("total_code_lines_accepted"),
})
ide_chat = entry.get("copilot_ide_chat", {})
ide_chat_id = uuid.uuid4()
copilot_ide_chats.append({
"copilot_ide_chat_id": ide_chat_id,
"global_id": global_id,
"total_engaged_users": ide_chat.get("total_engaged_users")
})
for editor in ide_chat.get("editors", []):
ide_chat_editor_id = uuid.uuid4()
copilot_ide_chat_editors.append({
"copilot_ide_chat_editor_id": ide_chat_editor_id,
"copilot_ide_chat_id": ide_chat_id,
"name": editor.get("name"),
"total_engaged_users": editor.get("total_engaged_users")
})
for model in editor.get("models", []):
ide_chat_editor_model_id = uuid.uuid4()
copilot_ide_chat_editor_models.append({
"copilot_ide_chat_editor_model_id": ide_chat_editor_model_id,
"copilot_ide_chat_editor_id": ide_chat_editor_id,
"name": model.get("name"),
"is_custom_model": model.get("is_custom_model"),
"custom_model_training_date": model.get("custom_model_training_date"),
"total_engaged_users": model.get("total_engaged_users"),
"total_chats": model.get("total_chats"),
"total_chat_insertion_events": model.get("total_chat_insertion_events"),
"total_chat_copy_events": model.get("total_chat_copy_events"),
})
dotcom_chat = entry.get("copilot_dotcom_chat", {})
dotcom_chat_id = uuid.uuid4()
copilot_dotcom_chats.append({
"copilot_dotcom_chat_id": dotcom_chat_id,
"global_id": global_id,
"total_engaged_users": dotcom_chat.get("total_engaged_users")
})
for model in dotcom_chat.get("models", []):
dotcom_chat_model_id = uuid.uuid4()
copilot_dotcom_chat_models.append({
"copilot_dotcom_chat_model_id": dotcom_chat_model_id,
"copilot_dotcom_chat_id": dotcom_chat_id,
"name": model.get("name"),
"is_custom_model": model.get("is_custom_model"),
"custom_model_training_date": model.get("custom_model_training_date"),
"total_engaged_users": model.get("total_engaged_users"),
"total_chats": model.get("total_chats")
})
配列にデータが入ったので、DataFrameを作成して、テーブルに追加させます。
データの型が暗黙値になっているので、明示的に定義することをおすすめします。
import pandas as pd
from pyspark.sql.types import StringType, StructField, DateType, IntegerType, StructType, BooleanType
columns = ['global_id', 'date', 'total_active_users', 'total_engaged_users']
df = pd.DataFrame(copilot_global_data, columns=columns)
df["global_id"] = df["global_id"].astype(str)
df["date"] = pd.to_datetime(df['date'])
schema = StructType([
StructField("global_id", StringType(), True),
StructField("date", DateType(), True),
StructField("total_active_users", IntegerType(), True),
StructField("total_engaged_users", IntegerType(), True),
])
spark_df = spark.createDataFrame(df, schema=schema)
spark_df.write.mode("append").format("delta").save("Tables/"+ "copilot_global_data")
columns = ['copilot_ide_completion_id', 'global_id','total_engaged_users']
df = pd.DataFrame(copilot_ide_completions, columns=columns)
df["global_id"] = df["global_id"].astype(str)
df["copilot_ide_completion_id"] = df["copilot_ide_completion_id"].astype(str)
schema = StructType([
StructField("copilot_ide_completion_id", StringType(), True),
StructField("global_id", StringType(), True),
StructField("total_engaged_users", IntegerType(), True),
])
spark_df = spark.createDataFrame(df, schema=schema)
spark_df.write.mode("append").format("delta").save("Tables/"+ "copilot_ide_completions")
columns = ['copilot_ide_completions_language_id', 'copilot_ide_completion_id', 'language', 'total_engaged_users']
df = pd.DataFrame(copilot_ide_completions_languages, columns=columns)
df["copilot_ide_completion_id"] = df["copilot_ide_completion_id"].astype(str)
df["copilot_ide_completions_language_id"] = df["copilot_ide_completions_language_id"].astype(str)
schema = StructType([
StructField("copilot_ide_completions_language_id", StringType(), True),
StructField("copilot_ide_completion_id", StringType(), True),
StructField("language", StringType(), True),
StructField("total_engaged_users", IntegerType(), True),
])
spark_df = spark.createDataFrame(df, schema=schema)
spark_df.write.mode("append").format("delta").save("Tables/"+ "copilot_ide_completions_languages")
columns = ['copilot_ide_completions_editor_id', 'copilot_ide_completion_id', 'editor', 'total_engaged_users']
df = pd.DataFrame(copilot_ide_completions_editors, columns=columns)
df["copilot_ide_completion_id"] = df["copilot_ide_completion_id"].astype(str)
df["copilot_ide_completions_editor_id"] = df["copilot_ide_completions_editor_id"].astype(str)
schema = StructType([
StructField("copilot_ide_completions_editor_id", StringType(), True),
StructField("copilot_ide_completion_id", StringType(), True),
StructField("editor", StringType(), True),
StructField("total_engaged_users", IntegerType(), True),
])
spark_df = spark.createDataFrame(df, schema=schema)
spark_df.write.mode("append").format("delta").save("Tables/"+ "copilot_ide_completions_editors")
columns = ['copilot_ide_completions_editor_model_id', 'copilot_ide_completions_editor_id', 'model', 'is_custom_model', 'custom_model_training_date', 'total_engaged_users']
df = pd.DataFrame(copilot_ide_completions_editor_models, columns=columns)
df["custom_model_training_date"] = pd.to_datetime(df['custom_model_training_date'])
df["copilot_ide_completions_editor_id"] = df["copilot_ide_completions_editor_id"].astype(str)
df["copilot_ide_completions_editor_model_id"] = df["copilot_ide_completions_editor_model_id"].astype(str)
df["is_custom_model"] = df["is_custom_model"].astype(bool)
schema = StructType([
StructField("copilot_ide_completions_editor_model_id", StringType(), True),
StructField("copilot_ide_completions_editor_id", StringType(), True),
StructField("model", StringType(), True),
StructField("is_custom_model", BooleanType(), True),
StructField("custom_model_training_date", DateType(), True),
StructField("total_engaged_users", IntegerType(), True),
])
spark_df = spark.createDataFrame(df, schema=schema)
spark_df.write.mode("append").format("delta").save("Tables/"+ "copilot_ide_completions_editor_models")
columns = ['copilot_ide_completions_editor_model_language_id', 'copilot_ide_completions_editor_model_id', 'language', 'total_engaged_users', 'total_code_suggestions', 'total_code_acceptances', 'total_code_lines_suggested', 'total_code_lines_accepted']
df = pd.DataFrame(copilot_ide_completions_editor_model_languages, columns=columns)
df["copilot_ide_completions_editor_model_language_id"] = df["copilot_ide_completions_editor_model_language_id"].astype(str)
df["copilot_ide_completions_editor_model_id"] = df["copilot_ide_completions_editor_model_id"].astype(str)
schema = StructType([
StructField("copilot_ide_completions_editor_model_language_id", StringType(), True),
StructField("copilot_ide_completions_editor_model_id", StringType(), True),
StructField("language", StringType(), True),
StructField("total_engaged_users", IntegerType(), True),
StructField("total_code_suggestions", IntegerType(), True),
StructField("total_code_acceptances", IntegerType(), True),
StructField("total_code_lines_suggested", IntegerType(), True),
StructField("total_code_lines_accepted", IntegerType(), True),
])
spark_df = spark.createDataFrame(df, schema=schema)
spark_df.write.mode("append").format("delta").save("Tables/"+ "copilot_ide_completions_editor_model_languages")
columns = ['copilot_ide_chat_id', 'global_id','total_engaged_users']
df = pd.DataFrame(copilot_ide_chats, columns=columns)
df["copilot_ide_chat_id"] = df["copilot_ide_chat_id"].astype(str)
df["global_id"] = df["global_id"].astype(str)
schema = StructType([
StructField("copilot_ide_chat_id", StringType(), True),
StructField("global_id", StringType(), True),
StructField("total_engaged_users", IntegerType(), True),
])
spark_df = spark.createDataFrame(df, schema=schema)
spark_df.write.mode("append").format("delta").save("Tables/"+ "copilot_ide_chats")
columns = ['copilot_ide_chat_editor_id', 'copilot_ide_chat_id', 'name', 'total_engaged_users']
df = pd.DataFrame(copilot_ide_chat_editors, columns=columns)
df["copilot_ide_chat_editor_id"] = df["copilot_ide_chat_editor_id"].astype(str)
df["copilot_ide_chat_id"] = df["copilot_ide_chat_id"].astype(str)
schema = StructType([
StructField("copilot_ide_chat_editor_id", StringType(), True),
StructField("copilot_ide_chat_id", StringType(), True),
StructField("name", StringType(), True),
StructField("total_engaged_users", IntegerType(), True),
])
spark_df = spark.createDataFrame(df, schema=schema)
spark_df.write.mode("append").format("delta").save("Tables/"+ "copilot_ide_chat_editors")
columns = ['copilot_ide_chat_editor_model_id', 'copilot_ide_chat_editor_id', 'name', 'is_custom_model', 'custom_model_training_date', 'total_engaged_users', 'total_chats', 'total_chat_insertion_events', 'total_chat_copy_events']
df = pd.DataFrame(copilot_ide_chat_editor_models, columns=columns)
df["custom_model_training_date"] = pd.to_datetime(df['custom_model_training_date'])
df["copilot_ide_chat_editor_model_id"] = df["copilot_ide_chat_editor_model_id"].astype(str)
df["copilot_ide_chat_editor_id"] = df["copilot_ide_chat_editor_id"].astype(str)
df["is_custom_model"] = df["is_custom_model"].astype(bool)
schema = StructType([
StructField("copilot_ide_chat_editor_model_id", StringType(), True),
StructField("copilot_ide_chat_editor_id", StringType(), True),
StructField("name", StringType(), True),
StructField("is_custom_model", BooleanType(), True),
StructField("custom_model_training_date", DateType(), True),
StructField("total_engaged_users", IntegerType(), True),
StructField("total_chats", IntegerType(), True),
StructField("total_chat_insertion_events", IntegerType(), True),
StructField("total_chat_copy_events", IntegerType(), True),
])
spark_df = spark.createDataFrame(df, schema=schema)
spark_df.write.mode("append").format("delta").save("Tables/"+ "copilot_ide_chat_editor_models")
columns = ['copilot_dotcom_chat_id', 'global_id','total_engaged_users']
df = pd.DataFrame(copilot_dotcom_chats, columns=columns)
df["global_id"] = df["global_id"].astype(str)
df["copilot_dotcom_chat_id"] = df["copilot_dotcom_chat_id"].astype(str)
schema = StructType([
StructField("copilot_dotcom_chat_id", StringType(), True),
StructField("global_id", StringType(), True),
StructField("total_engaged_users", IntegerType(), True),
])
spark_df = spark.createDataFrame(df, schema=schema)
spark_df.write.mode("overwrite").format("delta").save("Tables/"+ "copilot_dotcom_chats")
columns = ['copilot_dotcom_chat_model_id', 'copilot_dotcom_chat_id', 'name', 'is_custom_model', 'custom_model_training_date', 'total_engaged_users', 'total_chats']
df = pd.DataFrame(copilot_dotcom_chat_models, columns=columns)
df["copilot_dotcom_chat_model_id"] = df["copilot_dotcom_chat_model_id"].astype(str)
df["copilot_dotcom_chat_id"] = df["copilot_dotcom_chat_id"].astype(str)
df["custom_model_training_date"] = pd.to_datetime(df['custom_model_training_date'])
df["is_custom_model"] = df["is_custom_model"].astype(bool)
schema = StructType([
StructField("copilot_dotcom_chat_model_id", StringType(), True),
StructField("copilot_dotcom_chat_id", StringType(), True),
StructField("name", StringType(), True),
StructField("is_custom_model", BooleanType(), True),
StructField("custom_model_training_date", DateType(), True),
StructField("total_engaged_users", IntegerType(), True),
StructField("total_chats", IntegerType(), True),
])
spark_df = spark.createDataFrame(df, schema=schema)
spark_df.write.mode("append").format("delta").save("Tables/"+ "copilot_dotcom_chat_models")
レイクハウスを開いて、「新しいセマンティックモデル」を選択
先ほど作成したテーブルを全て選択します。
ここで作成したセマンティックモデルはDirectLake方式でデータの取り込みがされます。
以下のようにリレーションシップを作成しました。
Power BI Service(Web)でもいいのですが、テーマカラー設定ができないのでDesktopでやります。
「OneLakeカタログ」内の「Power BIのセマンティックモデル」を選択
先ほど作成したセマンティックモデルが出てくると思うので選択して接続
データが取り込めれました。
取得できる情報をもとにビジュアルが作れたので、Fabric環境にアップします。
右上の「発行」を選択
ワークスペースを選択して、アップロードが完了すると以下のようになります。
Power BIレポートがワークスペース内に追加されました。
これで一通り完了です。
以下のように可視化してみました。
弊社ではVScodeでPythonの利用が圧倒的に多いようです。
営業日(平日)は大体15~20人が使ってるのですが、まだまだ少ない気がするのでもっと増やしていきたいです。
ちょうど1年前にZennの取り組み状況を可視化するためにFabricを使い始めました。
↓これ
以前から大きく仕様が変わったことはないのですが以下は改善してくれるとより使いやすくなるなーと思いました。
これ何でできないんだ..
結構需要あると思うんだけどなー
現状はDesktop版じゃないとできません。
今回のケースは1日に1回データ更新すればいいので、ベストはImport方式です。
DirectLake方式が推奨されてるので今回も採用していますが、Import方式の方がレポートの読み込みが速くなるので、方式を選択できるようになるといいなーと。
ちなみにDataflow Gen2を使えばImport方式で取り込めれるのですが、Dataflow Gen2自体がものすごい容量を使ってしまうので中々手出しづらいです…
もしかしたら方法があるのかもですが、調べてみた限り分かりませんでした。
Notebook内のロジックは見えるけど、Access Tokenは他の社員に見えないようにしたいのですが、方法があるのかな?
Views: 0
Lea Verouはクラフトを助けました HTML 2023調査 – 最初は親切です! HTML、あなたは言いますか?何を尋ねるのですか? HTMLは、私が迅速に移動するテクノロジーと考えるものではありません。 あると聞いています
要素 今、それは新しいです。砂糖です
I actually do try to keep up with this sort of thing, and I’ll tell ya going through this survey had me clicking that “🤷 Never heard of it” choice quite a bit. Allow me to pick out a few that surprised me.
dateInput.showPicker()
。面白いねじれ、Codepenエディター内で試すことはできません。 HTMLInputElement::showPicker() called from cross-origin iframe.
エラー。うまくいきます ただし、デバッグモードで。できないと思います 宣言的に しかし、それを開きますよね?できるはずです。contenteditable
属性ですが、リッチな技術フォーマットをオプトアウトできるとは知りませんでした contenteditable="plaintext-only"
。 Firefoxはすでに持っていますが、みんなのように見えます。私についてすべてを作るために:ペンのヘッダー領域のUIを考えてください。所有してタイトルの上にホバリングする場合は、小さなアイコンをクリックして編集できます。私たちは使用しません contenteditable
誰かがYahoo!全体をコピーして貼り付けるのではないかと心配しているからですそこにホームページ(冗談、ちょっと)。しかし、豊かなテキストはそこではまったく関係ありません。これは、現在のようにテキストのためにテキストのためにテキストを使用したものに代わる素晴らしい代替品になるでしょう。name
複数の属性
要素は、それができるようにします open
一度に、一般的な「アコーディオン」パターン。私はどういうわけか、サファリがこれと一緒に最初にゲートから出るだろうと思った V17、 しかし、私は間違っていました。誰もそれを出荷していませんが、私はそれが好きです。発見するのが少し難しい場合、賢いアイデア。
のような属性 async
そして defer
しかし、本質的に反対を行うこれについては知りませんでした: blocking="render"
。それは素晴らしいパフォーマンスの特徴ではありませんが、ユーザーが何かを見る前に実際に実行する必要があるJavaScriptを持っている場合(何かを表示してレンダリング後にフロップするのではなく)、これが役立つことがわかります。 Reactを含むバンドルは、これを使用する必要があると思いますか?
3Dモデルを表示するための要素。どのように機能するのかわかりません。機能的ではなく、主にセマンティックだと思います。 早いようです。 import json from './foo.json' assert { type: 'json' };
それは儀式的なフェッチとパースから私を救うので、私は本当に好きです。 CSSバージョンALAについても聞いたことがあります import sheet from './styles.css' assert { type: 'css' };
それにより、これらのスタイルをドキュメントまたは耐性のあるシャドウルートにドンキングすることができます。しかし、私はそのアイデアがhtmlに拡張されていることを知りませんでした import { TabList } from "./tablist.html" with { type: 'html' }
; HTMLのその塊の中にあるカスタム要素を簡単に定義できるようになると思いますか?まだ想像できないので、例を見たいです。 focusgroup
属性。私はスミッジを読み上げました、そしてそれはとても面白いです!私がそれを正しく理解していれば、それは基本的にあなたがフォーカス可能な要素のグループを応答させることを可能にします 矢印キー グループ内のナビゲーション。タブをもう一度押すと、フォーカス可能な要素のグループ全体を残します。ラジオボタンのグループのように!しかし、あなたが望むどんなグループでも。 私たちはここのHTMLの土地に深く深く入っていますが、リンクの袋に手を伸ばして、最近保存したHTMLに関連する最も興味深いもののいくつかを共有してください。
HTMLに到着し始めている最も興味深いものの1つはポップオーバーです。 「ポップオーバーはウェブ上のどこにでもあります。」 Una Kravetsがそれらを紹介すると言います。 メニュー、ツールチップ、ボタンドロップダウンなど。これは、他のすべてのUIに座る必要があるUIの少し塊のようなものです。多くの場合、言うよりも簡単です! CSSのダーニング z-index
これまであなたを連れて行くことができます。ネストされたDOMに深い場合、多くの場合、ポップオーバーUIを少し高くすることができず、JavaScriptの操作に頼ってDOMのどこかに移動する必要があります。ネイティブのポップオーバーは、何があっても上にある魔法の高いレンダリングレイヤーに身を張っています。ひどく派手。
私は思うだろう アンカーポジショニング ピーナッツバターとゼリーのようにこれと一緒に行きます。真ん中にポップオーバーを鳴らしただけなら(デフォルトではそうだと思う)…それだけではありません ?幸いなことに、Hidde de Vriesが私たちをここでカバーしています ダイアログとポップオーバーは似ているようです。それらはどのように違うのですか? 私はできることを望みますが、私は彼の要約よりもうまくできないので:
さて、要約すると: モダリティ コンポーネントは、そのコンポーネントのみを使用できる状態です。何かがモーダルであるとき、他のすべてはそうです 不活性:アクセスから何らかの形でブロックされ、焦点が合い、通常は背景で不明瞭になります。何かモダルを作ることは実質的な決定であり、控えめに使用する必要があります。 ダイアログ モーダルまたは非モーダル(モードレスとも呼ばれます)にすることができます。
popover
Sは、トップレイヤーの存在、JSのないトググレアビリティ、ブラウザー提供された光解雇など、特定の動作と特性を備えた非モーダルダイアログを構築する新しい方法として、Open UIによって提案されています。とは異なり、a
popover
組み込みの役割がありません:開発者として、あなたはpopover
意味的に最も関連性の高い要素への属性
あなたは完全にサファリ17を知っています します 持っている?ポップオーバー。貼り付けます それらの例HTML ここで、Safari 17の新しいペンにコピーして貼り付けてテストを受けたい場合は、
Additional Information
Here’s something I wanted to tell you.
Rian Rietveldにちょっと5人 完璧なリンクを作成します。入門コンテンツはどこにでもありますが、良いものを見つけるのは難しいです(これは2021年です)。大量のコードの例ではありません。私にとっては、このような記事を書くのがさらに難しくなります。
私は先日、私がほとんど忘れていたリンク関連のトリックを少し使用しました: download
のような属性:
Download Pitch PDF
ここではデイブには良い点があると思います: マークダウン画像はパターンアンチパターンです。私はいつも言ってきました 画像は難しいです、一部には、あなたが知っていて、置く必要がある属性の数が原因
タグ。ただ loading="lazy"
一人で巨大です。 いくつかの Markdownプロセッサは、すべてではなく、Markdown構文で追加の属性を可能にします。ありがたいことに、MarkdownはHTMLをサポートしているので、それを使用してください。
私はあなたを残します HTMLレビュー:
2023年の問題は、デジタル文献と実験のモードにまたがる17の貢献で構成されています。詩的な楽器、インタラクティブなフィクション、イラスト入りのエッセイ、可動性の歌詞、言語庭園、ピクセル化された記憶があります。
Views: 0
Views: 0
LLM の機能を拡張するプロトコルとして MCP がある。(LSP みたいな感じ)
Ruby だと mcp-rb を使うことで実装が簡単にできる。
以下のように簡単な DSL で MCP サーバーが作れる。
require 'mcp-rb'
name 'local-mcp'
version '0.1.0'
resource "file://how-to-calculate-square" do
name "how-to-calculate-square"
description "Show how to calculate the square of a number"
call { "Use calculate-square tool" }
end
tool "calculate-square" do
description "Calculate the square of a number"
argument :number, Integer, required: true, description: "The number to be squared"
call do |args|
args[:number] * args[:number]
end
end
Claude, Cline, Copilot Agent など、様々なクライアントで MCP の対応が行われている。
bundler/inline
と併用するのがおすすめ利用する際には bundler/inline
と併用するのがおすすめ。
依存関係をファイル内に記述できるので、単体で実行しやすくなるのと、特定プロジェクト用の MCP サーバーを実装して、関係者に配るとかでは便利。
こんな感じで外部 API を叩くような MCP サーバーが簡単に書ける。
#!/usr/bin/env ruby
require 'bundler/inline'
gemfile do
source 'https://rubygems.org'
gem 'mcp-rb', require: 'mcp'
gem 'octokit'
end
name 'twitter-from-github'
version '0.1.0'
tool "twitter-from-github" do
description "Tell me about the twitter name of the user from GitHub status"
argument :username, String, required: true, description: "The name of GitHub user that you want to know the twitter name"
call do |args|
client = Octokit::Client.new
user = client.user(args[:username])
user.twitter_username
end
end
Views: 0
Amazonにて、KEEPTIMEの15.6インチモバイルモニターが特別価格で販売されている。セール価格は40%オフの8,939円。
本商品は、IPS液晶パネルを採用し、フルHDに対応する15.6インチモバイルモニター。178度の広い視野角を持ち、どの角度からでも鮮明な画像や映像を楽しめる。また、FreeSync低遅延技術が搭載され、テアリング現象を低減してよりスムーズなゲーム体験を実現する。重量は570gと軽量なので、持ち運びに便利。外出先でのサブモニターとしても使用できる。
なお、購入する際は、セール価格で販売されているかどうかを確認してから購入してほしい。
Views: 0